The expansion of the Internet of Things (IoT) requires new management methods for the mounds of data generated by IoT devices. As the increased use of Artificial Intelligence (AI) generates more data and clogs up the cloud, companies are looking to edge technology to decrease latency and maximize the use of AI and IoT.
Edge vs. Cloud
Both the edge and the cloud provide useful methods for data management. Understanding the differences between the two will help companies decide which method best suits their needs in different circumstances.
Edge Computing vs. Cloud Computing
Cloud computing stores and shares IT resources throughout a network, whereas edge computing collects information along the edge of a network. The edge and the cloud can communicate, but they can also function independently. Cloud computing relies on software, but edge computing relies on hardware. The cloud processes the data edge devices allow. That said, edge devices can also process information without accessing the cloud.
Join the AirFinder Difference!
- Innovation. Organizations can be freed up to innovate and bring more impactful products and services to market.
- Profitability. Increased profitability provides new opportunities to innovate and improve valuation.
- Digital Transformation. Discover competitive advantages, new revenue opportunities, improved customer relationships and increased efficiency.
Edge AI vs. Cloud AI
In the cloud, AI training takes place. AI training involves developing neural networks and algorithms to process data. This time-intensive process sorts mounds of data and shares within the network. AI inference takes place at the edge. AI inference involves using already developed algorithms and neural networks to process data collected at the edge. Because edge AI involves pre-trained algorithms, processing occurs almost immediately. Cloud AI is useful for managing new data and creating new neural networks, whereas edge AI is useful for fast response times and sifting through smaller amounts of data.
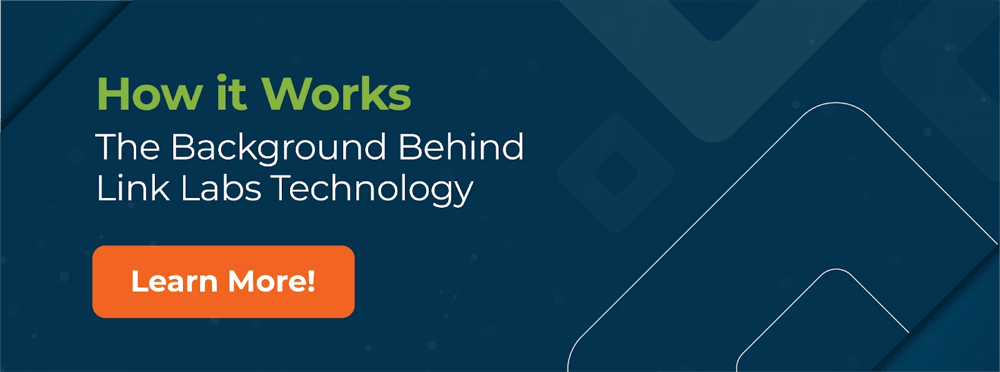
Edge AI Benefits
Decentralization
Because edge devices can work independently of the cloud, they do not need to rely on a network for communication. The edge device continues processing the data without interruption. In addition to uninterrupted processing, decentralization can provide another network security level. Proper encryption security can keep threats localized to edge devices rather than allowing them to reach the cloud. Although edge devices are decentralized, they can still communicate with the cloud when necessary, providing great flexibility in the data processing.
Real-Time Responses
Because edge computing minimizes the delay of sending information to the cloud for processing, edge AI employs inference in real-time. Edge AI relies on pre-trained algorithms to sift through data and provide an instant response, making it more effective in time-sensitive situations. If edge AI needs to be updated, the edge device can still communicate with the cloud to receive new neural networks and algorithms created during AI training.
Edge AI Uses
A massive issue in medical facilities is hoarding. Items, like wheelchairs, aren’t always in the location they’re supposed to be. This causes nurses stress since they have to search the hospital quickly to find one to use. Once they find one, they don’t want to return it, so they don’t have to spend time searching anymore, leading to a domino effect of other nurses hiding wheelchairs. AI technology can be used in tandem with RTLS to identify the location of wheelchairs and other vital items necessary for patient care. When RTLS is implemented, nurses no longer need to hoard wheelchairs because even if they aren’t left in the correct location, they can use the RTLS location to find out where an available wheelchair was left quickly and spend more time with their patient.
With the global smart manufacturing market expected to grow at a 12.4% CAGR until 2028, data solutions with high-speed adaptability are gr
owing faster. Higher processing speed and decentralization mean edge computing can respond to issues immediately. This speed makes it ideal for predictive maintenance, employee safety, and yield optimization in manufacturing. WIP can have unforeseen bottlenecks at times, delaying overall processes. With the use of AI, you can immediately be alerted of these bottlenecks so you can quickly address the issue and get back to work. Using RTLS for manufacturing lets you use Lean/Six Sigma principles for immediate feedback and response to bottlenecks. Instead of an after-action review or root cause analysis, operations managers can use real-time information to keep output flowing through the manufacturing process.
Within logistics, edge AI can be used to increase how efficiently products are transported between locations. With the help of this technology, companies can optimize operations by monitoring inventory levels and only sending out trucks when they are completely full and on schedule. Without AI technology, companies must resort to manual monitoring, leaving a risk for human error. The computing power of edge AI also lends itself to streamlining the arrival process between locations, allowing for automatic check-ins and docking availability updates. All these capabilities promote accountability for your staff and fill in communication gaps.
Closing Thoughts on AI at the Edge
AI gives you confidence that you will meet requirements and expectations on time. Our RTLS system, AirFinder, uses AI technology to enhance your existing processes. If you want to see how edge computing and RTLS work together, reach out for a demo.